Most ecommerce leaders don’t look at their revenue correctly.
With limited time to interpret their data, decision-makers rely on assumptions or instincts when making big decisions.
While there might be a monthly spreadsheet to reference or an agency update, this leaves a lot to be desired and ultimately can cost brands millions of dollars in missed opportunities.
The fastest-growing DTC brands today have a deep understanding of their data. They constantly run tests to maximize their revenue streams. If you’re an ecommerce leader in 2022, you need to understand which levers will drive the most revenue in the shortest amount of time.
So how can this be done in a practical way with limited resources?
In this Revenue Series by PenPath, we’ll explain how top-performing marketers interpret and use their ecommerce revenue data almost like a growth hack to scale sales. We’ll show you with detailed examples how exactly you can recreate this in your organization. Feel free to skip ahead:
Table of Contents
-
Where is Ecommerce Revenue Measured
-
Reviewing Your Platform’s Revenue
-
Growth Hack: Pairing Metrics With Revenue
-
Scaling Revenue Tactics
Review Your Platform’s Revenue
Platforms like Shopify, Woocommerce, and BigCommerce all handle orders and provide reliable revenue metrics. Pretty straightforward – these and other similar platforms are what we refer to when we say ecommerce platforms.
The tactics explained in this post can also be used with point-of-sale systems or third-party retailer platforms like Amazon since they contain similar data.
Here are a couple of screenshots of the Shopify and BigCommerce platforms and how they display revenue:
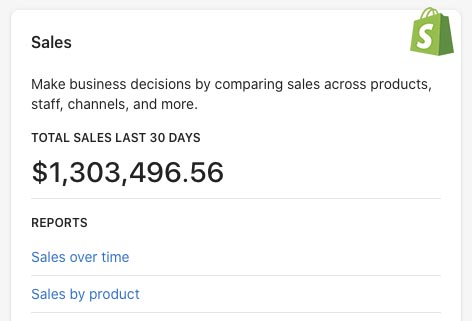
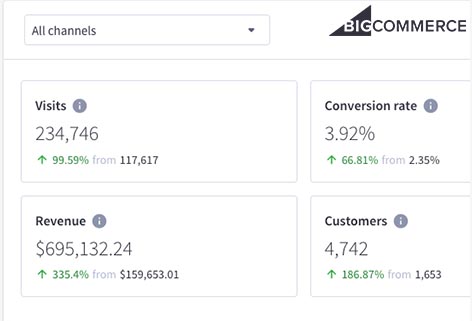
Growth Hack: Pairing Metrics With Revenue
To interpret your revenue, we encourage you to first be aware of the various ways revenue is calculated. Does your platform show Total Revenue? If so, does this exclude shipping, discounts, and taxes? Make sure you reference your platform’s documentation to confirm.
After clarifying what exactly “revenue” means, ask yourself, what other metrics could we pair with revenue to tell a more complete story?
Being fluent in your ability to pair metrics together means you can connect the dots. This is an absolute game-changer. Pairing metrics helps tell a more complete story so teams can understand what they need to do to improve performance — in this case, increase revenue.
Here’s an example of what we mean when we say pairing metrics together to tell a meaningful story:
The BlueShoe company understands they generated $560K in revenue from repeat customers in Q3. They also understand that returning customers love the Blue Jeans Jacket as it was the top seller for this segment (13% of returning customers purchased it).
Note how we explained revenue and then segmented this data further by pairing it with Repeat Customers and Product Sales.
This analysis tactic allows teams to essentially understand what levers might be available to influence sales. That makes data meaningful.
Now, how do you take something meaningful and make it actionable? In this simple example, you could assume that promoting Blue Jeans Jackets to new customers would generate more repeat purchase sales.
Relevant metrics you could pair with revenue:
- Revenue + LTV?
- Revenue + USA customers?
- Revenue + AOV?
- Revenue + New Customers?
Once you pair metrics, try to ask yourself “what does this mean?” (Meaningful) and “what do we need to do if we understand this?” (Actionable).
To further explain how you can do this, we wanted to show a couple of examples of this approach from beginning to end in more detail. Think of these as mini case studies.
Case Study: Scaling Ecommerce Revenue Tactics
Increasing how much new customers spend and how frequently they return
Recently, a PenPath customer — let’s call them BlueShoe — noticed a 33% drop in orders compared to the prior year. This was obviously hurting revenues. To turn things around, BlueShoe analyzed their data to find answers and a way out.
In this example, we’ll show how they used PenPath to complete this but this could have also been done with exported data and Excel, granted it would take a long time and significant data skills.
The two biggest opportunities they discovered were:
- Increase how much new customers are spending (AOV)
- Increase how often customers return to purchase (Repeat Purchase Rate)
The team concluded that these were their biggest opportunities to turn things around.
1) To increase AOV, BlueShoes identified the most popular products new customers purchased.
It turns out there are three products found in 25% of new customer purchases. Furthermore, these products had little volatility (low returns with predictable sales) and high demand (increasing orders every month).
The team then took this (meaningful) information and concluded that a 20% price increase would increase AOV and have little to no impact on total orders or conversion rates.
The BlueShoe team also took into account external factors such as increasing costs and inflation.
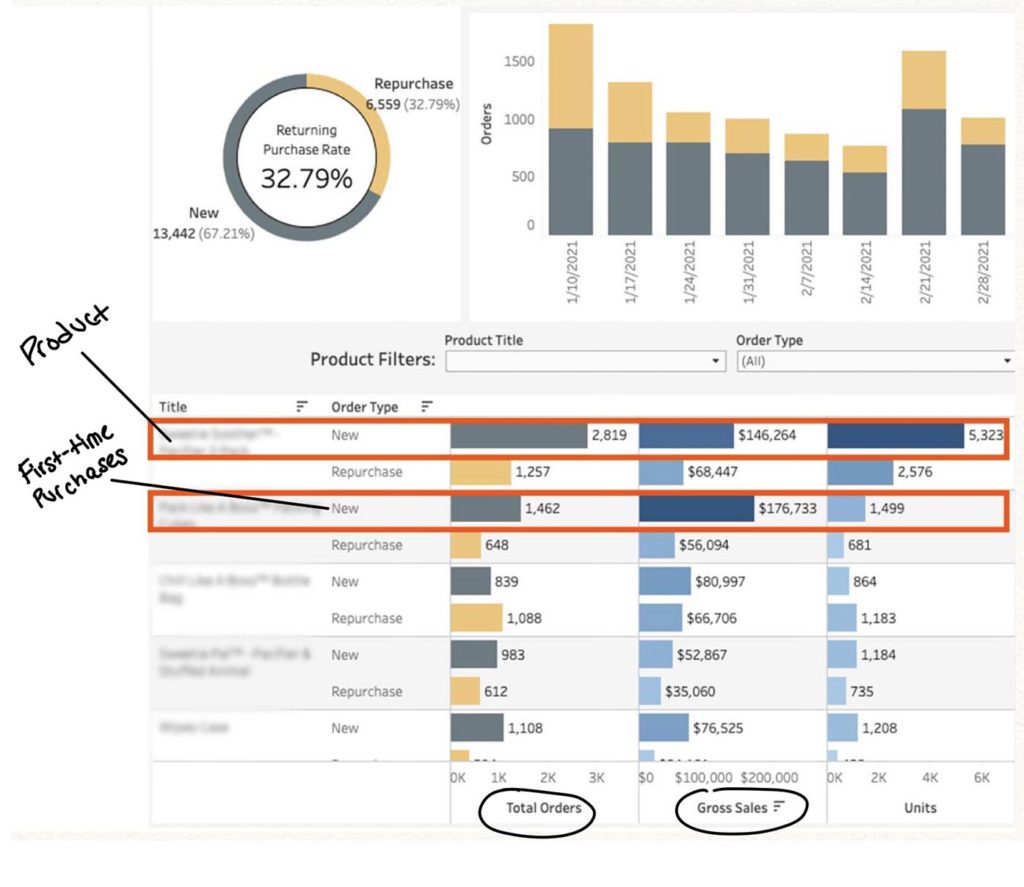
Using PenPath, BlueShoe identified which products were most frequently purchased by new customers.
When reviewing their data one month later, they compared their numbers with their prior-year performance. Here’s what they found:
- Total new customers increased by 4% (1,500)
- Orders for selected products decreased by 2% (450)
- AOV increased by $25 – from $65 to $90
- Total revenue from new customers increased from $46,000 to $55,200 — that’s a 20% increase in net new revenue
After seeing those numbers, the BlueShoe team concluded that their assumptions were correct and this test had clearly increased revenue. Increasing the price on high demand and low volatility products had virtually no impact on orders and increased sales by $9,200.
2) To increase how often customers returned to purchase, the BlueShoe team focused on products that sold well together.
First, the BlueShoe team identified popular repurchase products (see Repurchase row in the image above).
They discovered that a popular item for customers who returned to purchase was the Emerald Handbag.
Two insights surfaced after looking into this data further:
- Customers who purchased Emerald Handbag also commonly purchased the Leather Wallet
- Customers who purchased Emerald Handbag had a 45% higher lifetime value than the average customer
With this information, they were able to identify a lever that should lead to new streams of revenue.
The BlueShoe team identified customers who had purchased the Emerald Handbag without the Leather Wallet. This was a list size of about 2,300 customers — a sizable enough segment to run promotions against.
Their communications team was then able to focus their efforts on emails and retargeting this specific customer segment.
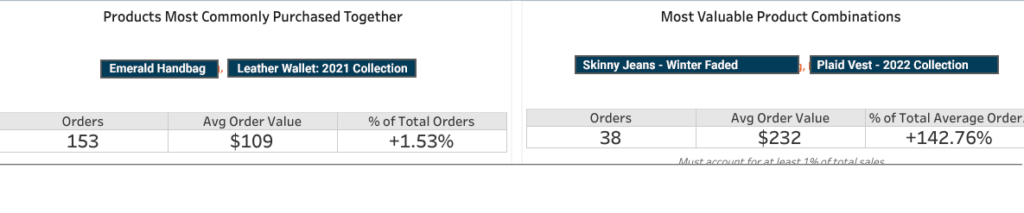
The above example shows how PenPath can highlight products that are commonly purchased together as well as the frequency and value.
When analyzing the results of this campaign, they noticed there was a 33% lift in Emerald Handbag sales compared to the same month last year. In one month, they were expecting to generate $10,000 in Emerald Bag sales but they actually generated $16,000 in sales. This was a $6,000 (60%) increase in revenue in the first month alone.
Results:
Making these two changes resulted in a total sales lift of $15,000. If those numbers are sustained, they would increase sales by about $150,000 in 12 months.
The takeaways going forward are to understand how to create data-informed initiatives and quickly test progress and results. Even if these tests did not work, your ability to track results and pivot could avoid wasting large amounts of money.
This interpretation of data can help decision-makers know what to do to increase sales.
Now that you understand how to interpret and use your ecommerce platform data to increase sales, let’s review where your paying customers are coming from and how they behave. For this, we will need Paid Media and Analytics platforms in this series.
Let us know if there are any metric/dimension combinations you’ve found to be effective!